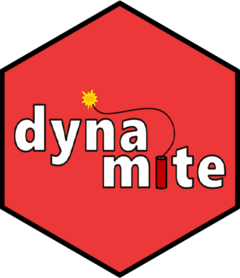
Extract Samples From a dynamitefit
Object as a Data Frame
Source: R/as_data_frame.R
as.data.frame.dynamitefit.Rd
Provides a data.frame
representation of the posterior samples of the model
parameters.
Usage
# S3 method for class 'dynamitefit'
as.data.frame(
x,
row.names = NULL,
optional = FALSE,
types = NULL,
parameters = NULL,
responses = NULL,
times = NULL,
groups = NULL,
summary = FALSE,
probs = c(0.05, 0.95),
include_fixed = TRUE,
...
)
Arguments
- x
[
dynamitefit
]
The model fit object.- row.names
Ignored.
- optional
Ignored.
- types
[
character()
]
Type(s) of the parameters for which the samples should be extracted. See details of possible values. Default is all values listed in details except spline coefficientsomega
. This argument is mutually exclusive withparameters
.- parameters
[
character()
]
Parameter(s) for which the samples should be extracted. Possible options can be found with functionget_parameter_names()
. Default is all parameters of specific type for all responses. This argument is mutually exclusive withtypes
.- responses
[
character()
]
Response(s) for which the samples should be extracted. Possible options are elements ofunique(x$priors$response)
, and the default is this entire vector. Ignored if the argumentparameters
is supplied.omega_alpha
, andomega_psi
. See alsoget_parameter_types()
.- times
[
double()
]
Time point(s) to keep. IfNULL
(the default), all time points are kept.- groups
[
character()
]
Group name(s) to keep. IfNULL
(the default), all groups are kept.- summary
[
logical(1)
]
IfTRUE
, returns posterior mean, standard deviation, and posterior quantiles (as defined by theprobs
argument) for all parameters. IfFALSE
(default), returns the posterior samples instead.- probs
[
numeric()
]
Quantiles of interest. Default isc(0.05, 0.95)
.- include_fixed
[
logical(1)
]
IfTRUE
(default), time-varying parameters for1:fixed
time points are included in the output asNA
values. IfFALSE
, fixed time points are omitted completely from the output.- ...
Ignored.
Value
A tibble
containing either samples or summary statistics of the
model parameters in a long format. For a wide format, see
as_draws.dynamitefit()
.
Details
The arguments responses
and types
can be used to extract only a subset
of the model parameters (i.e., only certain types of parameters related to a
certain response variable).
Potential values for the types
argument are:
alpha
Intercept terms (time-invariant or time-varying).beta
Time-invariant regression coefficients.cutpoint
Cutpoints for ordinal regression.delta
Time-varying regression coefficients.nu
Group-level random effects.lambda
Factor loadings.kappa
Contribution of the latent factor loadings in the total variation.psi
Latent factors.tau
Standard deviations of the spline coefficients ofdelta
.tau_alpha
Standard deviations of the spline coefficients of time-varyingalpha
.sigma_nu
Standard deviations of the random effectsnu
.corr_nu
Pairwise within-group correlations of random effectsnu
. Samples of the full correlation matrix can be extracted manually asrstan::extract(fit$stanfit, pars = "corr_matrix_nu")
if necessary.sigma_lambda
Standard deviations of the latent factor loadingslambda
.corr_psi
Pairwise correlations of the noise terms of the latent factors. Samples of the full correlation matrix can be extracted manually asrstan::extract(fit$stanfit, pars = "corr_matrix_psi")
if necessary.sigma
Standard deviations of Gaussian responses.corr
Pairwise correlations of multivariate Gaussian responses.phi
Describes various distributional parameters, such as:Dispersion parameter of the Negative Binomial distribution.
Shape parameter of the Gamma distribution.
Precision parameter of the Beta distribution.
Degrees of freedom of the Student t-distribution.
omega
Spline coefficients of the regression coefficientsdelta
.omega_alpha
Spline coefficients of time-varyingalpha
.omega_psi
Spline coefficients of the latent factorspsi
. Note that in case ofnonzero_lambda = FALSE
, mean of these are used to flip the sign ofpsi
to avoid multimodality due to sign-switching, butomega_psi
variables are not modified.zeta
Total variation of latent factors, i.e., the sum ofsigma_lambda
andtau_psi
.
See also
Model outputs
as.data.table.dynamitefit()
,
as_draws_df.dynamitefit()
,
coef.dynamitefit()
,
confint.dynamitefit()
,
dynamite()
,
get_code()
,
get_data()
,
get_parameter_dims()
,
get_parameter_names()
,
get_parameter_types()
,
ndraws.dynamitefit()
,
nobs.dynamitefit()
Examples
data.table::setDTthreads(1) # For CRAN
as.data.frame(
gaussian_example_fit,
responses = "y",
types = "beta"
)
#> # A tibble: 200 × 10
#> parameter value time category group response type .draw .iteration .chain
#> <chr> <dbl> <int> <chr> <int> <chr> <chr> <int> <int> <int>
#> 1 beta_y_z 1.96 NA NA NA y beta 1 1 1
#> 2 beta_y_z 1.97 NA NA NA y beta 2 2 1
#> 3 beta_y_z 1.96 NA NA NA y beta 3 3 1
#> 4 beta_y_z 1.95 NA NA NA y beta 4 4 1
#> 5 beta_y_z 1.96 NA NA NA y beta 5 5 1
#> 6 beta_y_z 1.97 NA NA NA y beta 6 6 1
#> 7 beta_y_z 1.96 NA NA NA y beta 7 7 1
#> 8 beta_y_z 1.96 NA NA NA y beta 8 8 1
#> 9 beta_y_z 1.97 NA NA NA y beta 9 9 1
#> 10 beta_y_z 1.95 NA NA NA y beta 10 10 1
#> # ℹ 190 more rows
# Basic summaries can be obtained automatically with summary = TRUE
as.data.frame(
gaussian_example_fit,
responses = "y",
types = "beta",
summary = TRUE
)
#> # A tibble: 1 × 10
#> parameter mean sd q5 q95 time group category response type
#> <chr> <dbl> <dbl> <dbl> <dbl> <int> <int> <chr> <chr> <chr>
#> 1 beta_y_z 1.97 0.0112 1.95 1.98 NA NA NA y beta
# Time-varying coefficients "delta"
as.data.frame(
gaussian_example_fit,
responses = "y",
types = "delta",
summary = TRUE
)
#> # A tibble: 60 × 10
#> parameter mean sd q5 q95 time group category response type
#> <chr> <dbl> <dbl> <dbl> <dbl> <int> <int> <chr> <chr> <chr>
#> 1 delta_y_x NA NA NA NA 1 NA NA y delta
#> 2 delta_y_x -0.161 0.0299 -0.214 -0.110 2 NA NA y delta
#> 3 delta_y_x -0.909 0.0351 -0.966 -0.852 3 NA NA y delta
#> 4 delta_y_x -0.882 0.0253 -0.924 -0.839 4 NA NA y delta
#> 5 delta_y_x -0.916 0.0217 -0.959 -0.884 5 NA NA y delta
#> 6 delta_y_x -1.06 0.0202 -1.10 -1.03 6 NA NA y delta
#> 7 delta_y_x -1.14 0.0224 -1.18 -1.10 7 NA NA y delta
#> 8 delta_y_x -0.997 0.0226 -1.04 -0.962 8 NA NA y delta
#> 9 delta_y_x -0.902 0.0248 -0.942 -0.864 9 NA NA y delta
#> 10 delta_y_x -1.01 0.0223 -1.05 -0.975 10 NA NA y delta
#> # ℹ 50 more rows
# Obtain summaries for a specific parameters
as.data.frame(
gaussian_example_fit,
parameters = c("tau_y_x", "sigma_y"),
summary = TRUE
)
#> # A tibble: 2 × 10
#> parameter mean sd q5 q95 time group category response type
#> <chr> <dbl> <dbl> <dbl> <dbl> <int> <int> <chr> <chr> <chr>
#> 1 tau_y_x 0.364 0.0770 0.253 0.497 NA NA NA y tau
#> 2 sigma_y 0.198 0.00404 0.192 0.205 NA NA NA y sigma